The use of analytics — using data and statistics to better understand something — is growing across most sports. This is especially true in soccer, where the most successful teams are also frequently the most dedicated to analytics. In MLS, Toronto FC and the Seattle Sounders, the two teams responsible for 3 of the last 4 MLS Cup matchups, are both at the forefront of the industry.
This piece, ahead of the 2016 MLS Cup, outlines their commitment to data-driven decision-making, and they’ve both doubled down on it since then. The Sounders hosted a two-day conference on analytics this past summer, and Toronto have been open about their use of data recently, as well. Outside of MLS, Barcelona, Liverpool and Bayern Munich have all been public about their willingness to use data to inform decision-making. In the international game, so have U.S. Soccer and the German Football Association. More and more, winning teams’ decision makers have an analytical edge over the competition.
The goal of this piece is to give you a guide and a way in, including who to follow, where to look, and what to know about analytics in MLS.
Analytics doesn't replace traditional scouting
Though analytics in soccer is relatively new, it doesn’t negate or supersede more traditional methods of evaluation. Video analysis and traditional scouting, or the “eye test”, are still important.
Ideally, the three are complementary — all are just different types of data. The best clubs seamlessly implement analytics to work with those other methods, by understanding the strengths and weaknesses of each. When
LAFC watch game tape of Leon to prep for CCL, for example, stats can help pick out games Leon has played against teams with a pressing style most similar to LAFC’s.
Analytics should be tied closely to an understanding of the game
Much of analytics is really about putting concepts that soccer people already understand into terms that can be described with data. Most people know intuitively what a counterattack is, for example. A goal of analytics, though, is to answer a question like “How valuable is
Real Salt Lake’s ability to prevent counterattacks?” To do that, we need to define and quantify a “counterattack” first. From that definition, an analyst can determine that RSL’s defensive ability there prevented up to five or six goals in 2019. The goal of this quantification is to give an analyst a way to describe at scale what happens on the field.
Team Expected Goals
Not all shots are equal — a shot closer to the goal is obviously more valuable than a shot farther from goal.
Expected goals (xG) puts a number to the quality of a shot. A shot that has a 50% chance of going in has an xG of 0.5. A shot with a 10% chance of going in has an xG of 0.1. Adding up the xG of the shots a team takes is their “xG for” (xGF), and adding up the xG of the shots the team allows is their “xG against” (xGA). xGF minus xGA equals the “xG difference”, or xGD. Each data provider has their own formula for actually calculating xG, but all typically include information like the distance from and angle to goal, and what sort of scenario the shot came from (a cross or a set piece, for example).
Expected goals are a widespread and important metric in analytics. Teams that get more and better shots than their opponents tend to perform well in the long run, even if those shots don’t always go in, so xGD can be a better measure of team strength than just goal difference. 2019 LAFC were the most dominant team in MLS since 2011 according to xGD.
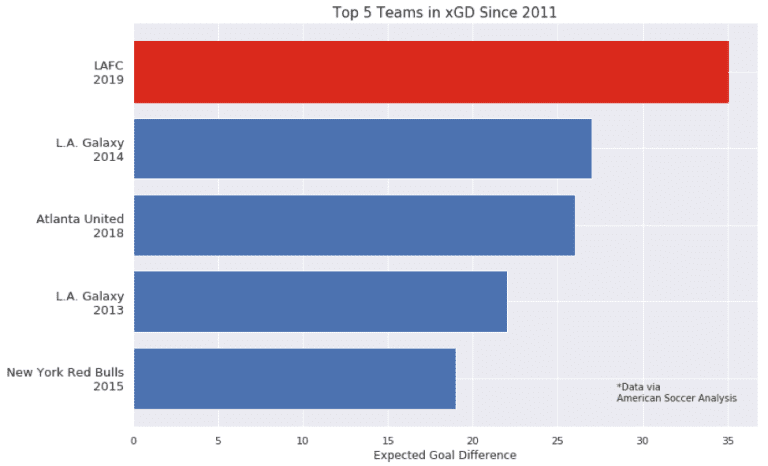
Player Expected Goals
Players that get many high quality shots tend to score lots of goals. A player’s ability to get shots from good locations is actually a more important factor in scoring than his ability to finish chances from any location. Though analytics frequently confirm what people within soccer intuitively know, this is one example where statistics contradicts prevailing soccer wisdom.
Goalkeeper Expected Goals
For goalkeepers, xG relates to how difficult a save is. A keeper’s “goals saved above expected” measures shot-stopping ability — how many more goals did a keeper save than an average keeper would have. Matt Turner was historically excellent at that stat in 2019:
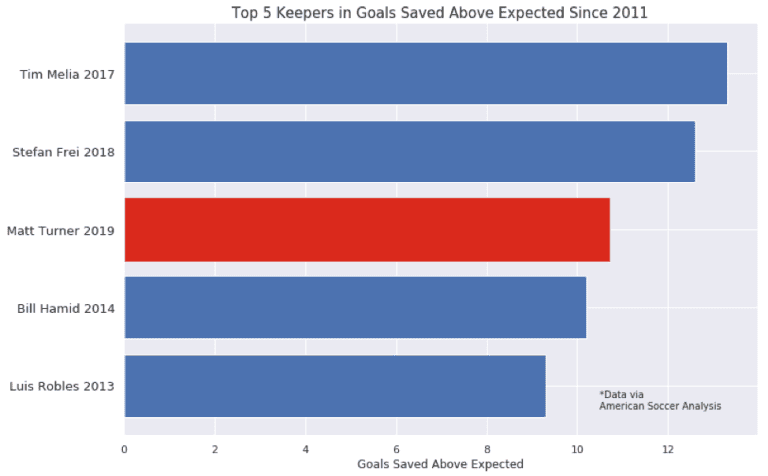
Possession value
Expected goals are a good measure for the chances that a team creates and concedes, but they’re only recorded when a team takes a shot. Possession value, though, calculates the probability of a goal being scored at any point in a possession. Just like with shots, a team on the ball right outside the box is much more dangerous than a team knocking it around in its own half — this is why straight percentage of possession stats can be misleading. Possession value models that danger.
Much of analytics focuses on determining the actions that lead to higher and lower value possessions. The Sounders last season, for example, were incredibly effective in attacking in transition down the left. Possession value provides a framework for evaluating the ways teams play, and it has led to interesting analyses of different parts of the game.
Defensive actions
Unlike offensive ability, defensive ability is incredibly difficult to evaluate from a possession value framework. Ike Opara is an illustrative example. He was Defender of the Year last season, but made just 1.5 tackles per 90, 22nd most in the league among center backs. Instead, his positioning was so good that he didn’t need to make many plays on the ball. That contribution is difficult to value with data that only includes on-ball actions.
Soccer analytics, instead, is much better about describing defenses. We can say that Opara in 2019 was only moderately aggressive in stepping to the ball. We can look at a map of his tackles and interceptions to say where he was more or less aggressive (more yellow means more actions in those areas) and on what type of possessions:
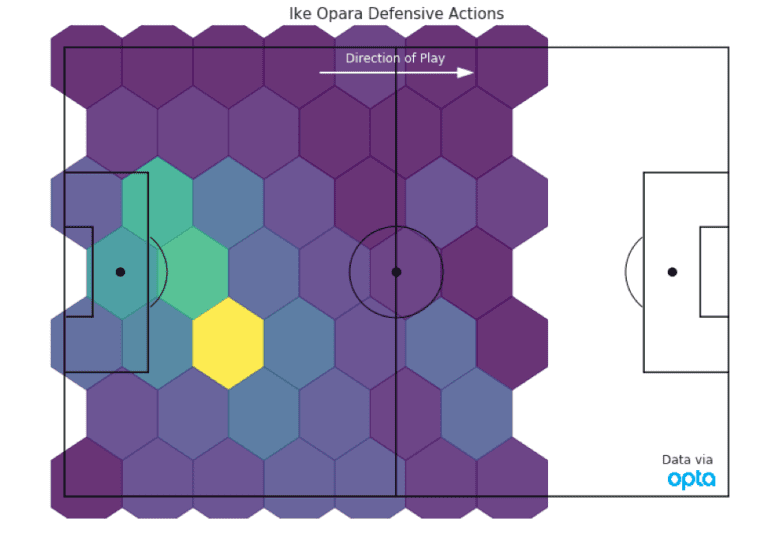
It’s harder to say, though, what the true value of that of aggressiveness was.
Generally, defensive statistics are adjusted for possession. A team with less possession has more opportunities to make defensive plays, so measures of their actions are adjusted accordingly. Passes per defensive action (PPDA) is one popular metric to measure the extent of a team’s press. Pressure on the ball is also used. Looking at where a team chooses to pressure the ball or make tackles and interceptions can be used to describe a team’s defensive set up: Are they a low block or high block? Do they press out wide or in the middle of the field?
Passing ability
Similar to measuring the quality of a shot (with xG), analytics can model the difficulty of a pass. Completing a pass into an opponent’s six-yard box is much more difficult than hitting a pass between two center backs, for example. Passing scores measure which players are able to hit passes at rates above what would be expected.
Game states
“Game state” is a catch-all term for the condition of the game — is a team winning, tied, or trailing? Are they home or away? Are they a man up or a man down? Game state effects provides important context for understanding statistics. Home-field advantage in MLS is very strong, and home teams take more shots, score more goals and win more often.
As a result, a team that starts the season with two months of road games (like Portland or D.C. United in the last two years) will look worse relative to the rest of the league. Teams on the road tend to play with a deeper defensive line and less possession, so stylistically, they might look like they play in a very deep block for those first two months.
Visualizations
Data visualization can also fall under the domain of analytics. Here are some popular ones:
- xG maps show the value and location of the shots a team or player creates or concedes, either within a game or across a season.
- Passing networks describe passing connections — who passes to who, and where — in order to understand how a team plays.
- Radars and bar charts display a team’s or player’s performance across different metrics.
- Pass sonars describe the passing tendencies and abilities of players.
Companies collecting data
The increasing availability and amount of data captured around the sport spurs much of analytics’ growth. Opta, the official data partner of MLS, tracks what is known as “event data” for every single MLS game. Their trackers record every action that takes place on the ball, and where on the field that action occurs. Companies like Second Spectrum, SportLogiq, and Metrica Sports (which counts the Galaxy, Seattle and LAFC among its clients) collect “tracking data” that describes the locations of the ball and every player on the field, multiple times per second. Stat Sports and Catapult use wearables to record performance data, on things like a player’s heart rate and distance ran. These technologies all produce data that can be parsed, transformed, analyzed, and disseminated.
Statistics sites
Stats sites use the data produced by the companies above to provide statistics and metrics. Tracking data metrics are mostly not public, currently.
- FBRef: Football Reference is the soccer site of the Sports Reference family.
- WhoScored: WhoScored offers player grades based on data
- FiveThirtyEight: 538 provides season and per-game predictions, modeled on top of shot based and non-shot based xG calculations
- American Soccer Analysis: ASA (of which I am a member) is the foremost site for analytics within MLS, and it includes advanced metrics for MLS back to 2011
- SmarterScout: a fantastic subscription service that includes a free tier for fans
Analysis sites
The sites listed here use advanced stats and metrics to analyze different aspects of the game.
- American Soccer Analysis: ASA also includes articles analyzing American soccer
- Statsbomb: Statsbomb is an event data provider, with a blog covering many different leagues including MLS
- Opta blog: Opta’s blog often provides write-ups on their latest product iterations. This piece on identifying phases of play is an excellent example of the process of moving from an intuitive concept into a clear data definition.
Analytics writers
Twitter is generally the best place to keep up with new analytics work. These are some writers who tend to write specifically about analytics.
Writers who use stats
These writers approach the game from a holistic perspective, and frequently incorporate analytics into their work. (Here is a great example).
Where analytics are headed
Soccer analytics is still a fairly new field, but it already has a large impact on MLS. It has influenced changes in how the game is played — teams shoot closer to goal and take shorter goal kicks and quicker throw-ins, all of which is suggested by looking at data. As the amount of data available to teams increases, they will get more efficient in how they play, especially defensively, where analytics currently has a blindspot.
Within front offices, analytics is changing how teams operate. More and more teams are making stats hires, and the teams that already employ analytics people are building out their departments. It seems reasonable to expect that, as in baseball and basketball, before too long some teams will be run at the top by a stats person. And in the media, coverage of the league often is driven by stats-based narratives. FOX Sports commentator John Strong quotes xG on broadcasts, and many writers are comfortable using it. That coverage will get more accurate and more widespread as fans get more familiar with it.
Analytics influences the league in many ways, and that influence is going to continue to grow in MLS.
Kevin Minkus is a data scientist living in Philadelphia. He has been writing about and consulting in soccer analytics since 2015.